Synthetic Data Sorces
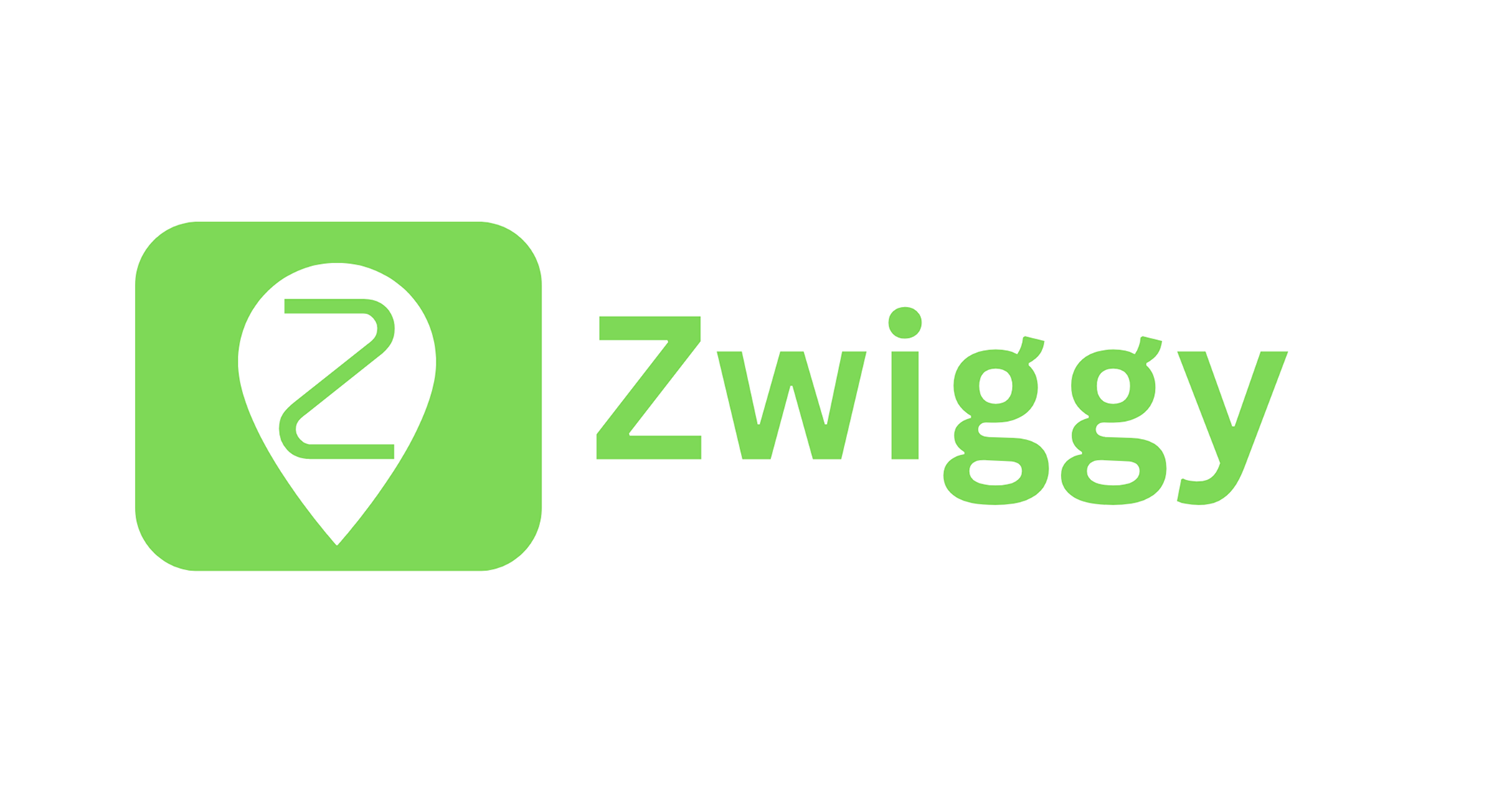
Zwiggy is a synthetic, full-scale dataset designed to simulate the operations of a modern food delivery platform, catering to data professionals, engineers, and learners. It covers key components such as user profiles, restaurant menus, orders, deliveries, and payments, enabling hands-on practice with real-world scenarios in a privacy-compliant format. Zwiggy is ideal for training ML models, testing backend systems, and analyzing operational data, while also serving as a resource for SQL interviews, database tasks, and ETL pipeline development. With its relational structure, timestamped events, and geospatial data, it offers a reliable foundation for enterprise-grade testing and exploration.
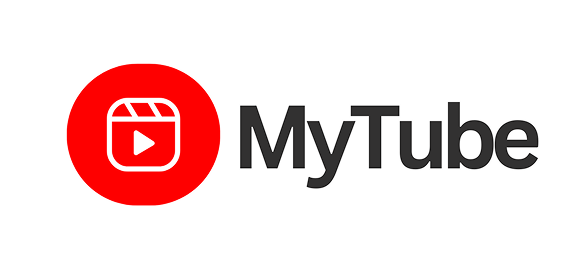
MyTube is a synthetic dataset that simulates the ecosystem of a large-scale video streaming platform, offering a realistic environment for modeling user behavior, analyzing engagement, and experimenting with recommendation and monetization strategies. It captures key platform elements such as users, video uploads, subscriptions, comments, likes/dislikes, watch histories, and ad interactions. Ideal for machine learning engineers, data scientists, and backend developers, MyTube supports features like content ranking, viewer retention, and personalized feeds. It's a valuable resource for practicing SQL queries, building ETL pipelines, and experimenting with content moderation and monetization in a safe, privacy-compliant format.

Musify is a synthetic dataset that replicates the core functions of a modern music streaming platform, including user activity, audio content, playlists, and recommendation dynamics. Designed for data scientists, machine learning engineers, and developers, it provides a structured environment for exploring audio data, modeling user preferences, and building recommendation engines. The dataset includes users, songs, artists, albums, playlists, and user interactions like likes and skips, supporting applications such as collaborative filtering, user segmentation, and content recommendation. It's also ideal for technical interviews, data pipeline construction, and simulating backend systems for music-based applications in a privacy-compliant format.
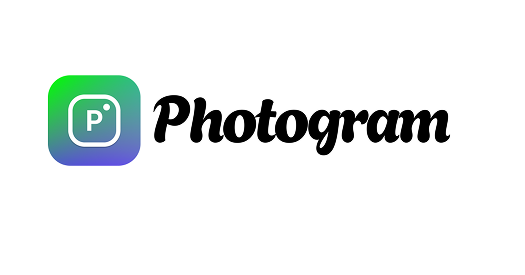
Photogram is a synthetic dataset simulating a modern image-sharing social media platform, designed for data science, software development, and analytics. It includes data on users, posts, likes, comments, follows, hashtags, and media metadata, making it ideal for training models, testing content recommendation algorithms, and simulating social media workflows. With realistic engagement patterns and interaction timelines, it supports use cases like content performance analysis, influence modeling, and backend feature validation. Photogram is also a valuable resource for students and engineers preparing for roles in social media or content-focused applications.
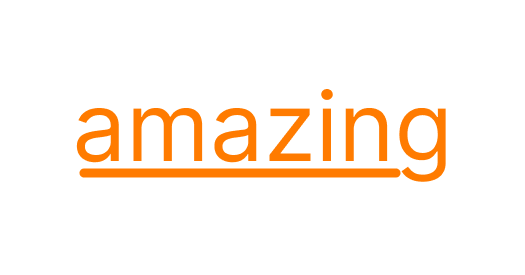
Amazing is a synthetic dataset simulating a large-scale e-commerce platform, covering everything from product listings and customer profiles to orders, payments, and post-purchase interactions. It provides a structured environment for developing recommendation engines, sales forecasting models, fraud detection systems, and more. With detailed data on customers, vendors, products, reviews, and order histories, it supports backend development, retail analytics, and business insights. Ideal for students and professionals in e-commerce, Amazing offers hands-on practice in database design, ETL development, and advanced SQL querying, making it a versatile resource for data science and analytics.
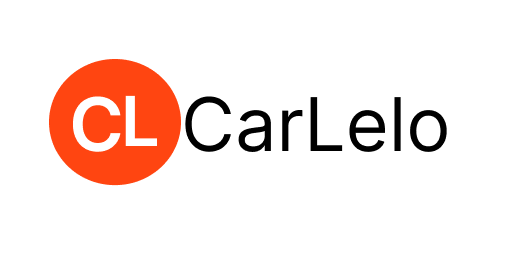
Carlelo is a synthetic dataset designed to simulate a car listing and sales platform, featuring data on vehicle listings, buyer interactions, and pricing trends. It supports price prediction, customer preference analysis, and backend testing, with records on users, vehicles, transactions, dealerships, reviews, and pricing. Ideal for machine learning, developers, and analysts, Carlelo enables the creation of predictive models for price optimization and demand forecasting, simulates backend workflows, and explores market trends and buyer behavior. It's also a valuable resource for SQL practice and cohort analysis in the automotive sales sector.
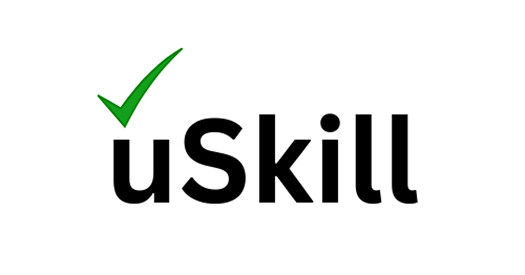
uSkill is a synthetic dataset simulating an online learning platform, offering data on user behavior, course effectiveness, and platform performance. It includes records for users, courses, instructors, enrollments, quizzes, and feedback, enabling analysis of the full learning lifecycle. Ideal for machine learning engineers, developers, and analysts, uSkill supports projects like course recommendations, churn prediction, user dashboard testing, and cohort analysis. It's also a valuable resource for SQL practice and building ETL pipelines in the educational sector.
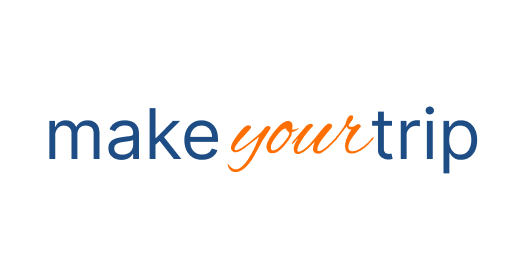
MakeYourTrip is a synthetic dataset simulating a travel booking platform, covering flight reservations, hotel bookings, customer preferences, and transactions. It includes data on users, flights, hotels, bookings, payments, and reviews, offering insights for modeling user behavior, predicting travel trends, and optimizing booking systems. Ideal for machine learning, developers, and analysts, MakeYourTrip supports projects like recommendation models, booking pattern analysis, price optimization, and backend testing. It's also useful for SQL practice, ETL processes, and analyzing market demand and customer behavior.
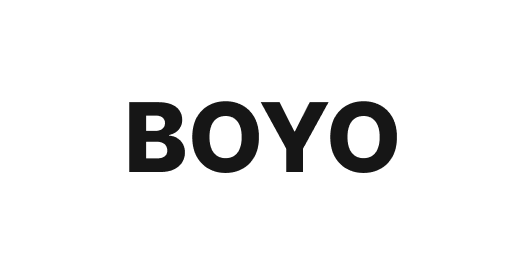
BOYO is a synthetic dataset simulating a digital hospitality booking platform, covering the entire guest journey from property discovery to booking, check-in, and feedback. It includes structured data on users, properties, bookings, pricing, payments, reviews, and location metadata, making it ideal for analyzing guest behavior, building dynamic pricing models, and simulating booking workflows. Suitable for machine learning projects, backend testing, and data analysis, BOYO supports cancellation prediction, personalized recommendations, and demand forecasting. It's also valuable for SQL practice, relational modeling, and ETL processing.
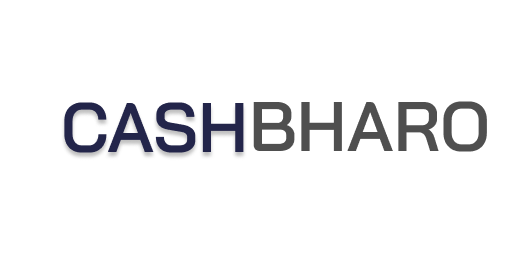
CashBharo is a synthetic dataset that replicates a cashback and affiliate marketing platform, capturing user interactions with offers, purchases, referrals, and campaigns. It includes data on users, merchants, transactions, cashback offers, and campaign performance, making it ideal for building transaction tracking systems, optimizing campaigns, and analyzing customer behavior. This dataset supports machine learning projects for personalized recommendations, fraud detection, and redemption predictions, while developers can test affiliate tracking and payment workflows. It also provides valuable insights for analysts studying promotional effectiveness, customer segmentation, and offer conversions.
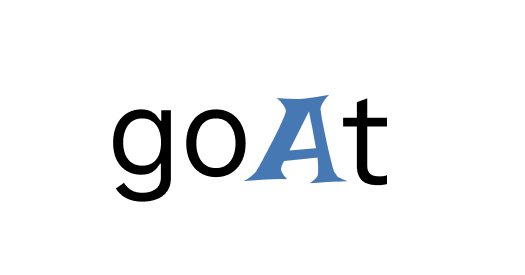
GOAT is a synthetic dataset that mirrors the ecosystem of a direct-to-consumer electronics brand, covering categories like audio gear, wearables, and smart accessories. It includes data on users, product listings, transactions, inventory, reviews, and support tickets, making it ideal for analyzing product performance, consumer preferences, and marketing effectiveness. Suitable for machine learning, development, and analytics, GOAT supports use cases such as demand forecasting, A/B testing, and customer service automation. It helps model customer lifetime value, predict returns, and personalize recommendations while offering valuable insights for cohort behavior and sales trends.
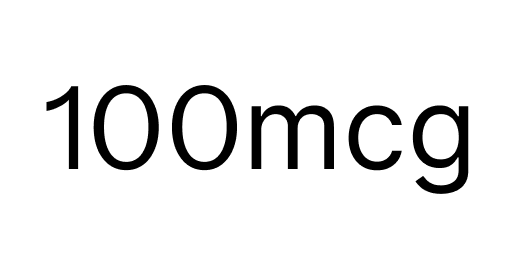
100mcg is a synthetic dataset that simulates an online healthcare and pharmacy platform, including pharmaceutical product listings, user purchases, medicine interactions, and customer feedback. It features data on users, products, medications, prescriptions, orders, and reviews, making it ideal for building predictive models, recommendation systems, and pricing optimization. Data scientists can analyze customer buying behavior, while developers can simulate order fulfillment and prescription validation. Analysts can explore sales trends, product demand, and marketing strategies, making it a valuable resource for SQL practice, cohort analysis, and A/B testing.